A Computer ‘Game’ to Identify Opioid Abuse Patients at High Risk of Relapse
A Computer ‘Game’ to Identify Opioid Abuse Patients at High Risk of Relapse
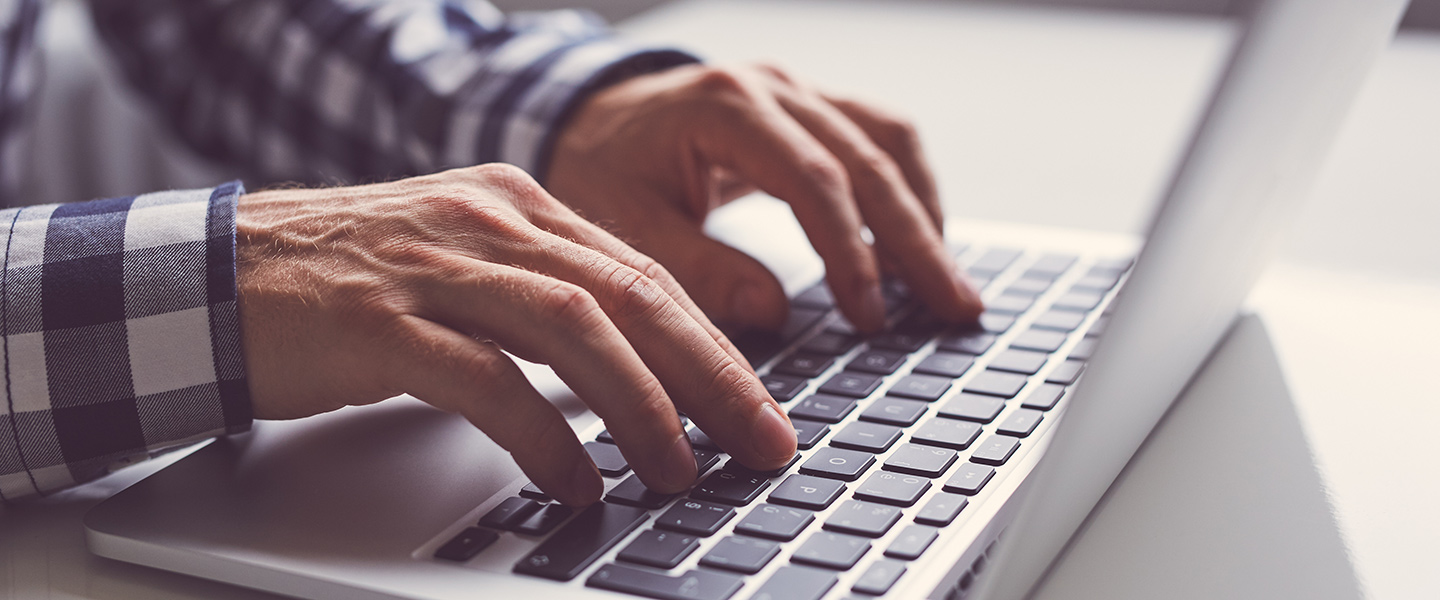
Researchers have tested a game-like computer program that in a clinical study provided a means of determining which patients in an opioid-treatment program were highly vulnerable to relapse in the coming week.
Drug overdose is now the leading cause of unintentional death in the U.S., with opioids including heroin and fentanyl accounting for the largest portion of these incidents. An estimated 2 million Americans are thought to have opioid use disorder (OUD), and while treatments to overcome it are well established, patients in treatment programs have high rates of reuse, relapse, and dropout.
Standard treatment often involves psychotherapy plus treatment with medications such as methadone, naloxone, or buprenorphine, which help patients get over their cravings while not producing a “high.” Yet a majority of patients in treatment use opioids again at some point during their program, and over half relapse within a year.
With this fact in mind, a team of researchers at New York University conducted a study using tools from the relatively new fields of computational psychiatry and decision neuroscience. Their aim was to discover and try to measure specific cognitive factors that might enable doctors to identify which of their patients are at highest risk of relapse at any given point during treatment.
A series of experiments was conducted over 7 months, led by 2016 BBRF Young Investigator Anna Konova, Ph.D., and Silvia Lopez-Guzman, M.D., Ph.D. They demonstrated that periodic measurements of patients’ tolerance for risk—which fluctuates over time—were directly correlated with the odds that they would re-use opioids during the following 7 days. The study’s senior investigator, Paul Glimcher, Ph.D., is a neuro-economist who has pioneered decision-making analysis in animals and people.
The tool used in the newly reported study is a kind of computer game that tests patients’ willingness to take a financial risk. Specifically, it was designed to distinguish their willingness to take a known risk—one whose probability of success is set forth in advance—versus a risk whose likelihood of “paying off” was ambiguous. Three levels of ambiguity were tested, corresponding with great, moderate, and slight risk.
The test subjects included 70 patients, on average in their mid-40s, undergoing treatment for opioid use disorder. They were joined in the study by 50 matched control subjects without addictions, who were given the same “risk-tolerance” tests for comparative purposes over the same 7 months, although not as frequently. The test was given repeatedly over time, a key factor in the study since the researchers were interested in discovering a way to measure tolerance for risk as it changed from week to week and month to month. A daily readout would have been preferable, the team said, but was not within the scope of the present study.
The researchers reported that it was possible to measure changes in patients’ risk behavior over the weeks, and that higher tolerance for ambiguity on patients’ part in any given test correlated with a marked increase in the likelihood that that patient would reuse an opioid during the next 7 days.
Risk tolerance has long been thought to be a potential criterion for identifying those at high risk for relapse, but until this study it wasn’t clear if the degree of uncertainty in risk-taking was the distinguishing factor. Although tolerance for known risks is thought to be stable over time, and one of the “traits” associated with drug-taking, measuring it did not deliver short-term signals in this study that could be used to monitor the effectiveness of treatment.
In contrast, only tolerance for ambiguous risk fluctuated over time. A sharp spike in tolerance for unknown or hard-to-gauge risks correlated with a substantial increase in the likelihood that a patient would use an opioid in the coming days.
The team said that this information, if validated in future studies, might be combined with other measures of clinical assessment of patients in opioid treatment programs, such as those that measure levels of a patient’s craving. A future direction, the team said, is to deploy such measures alongside other assessments on mobile platforms such as smartphones. This would permit the team to track “risk states” of patients more dynamically, and to use the information provided in their clinical care.