Using Brainwave Patterns to Predict and Understand Psychiatric Disorders
Using Brainwave Patterns to Predict and Understand Psychiatric Disorders
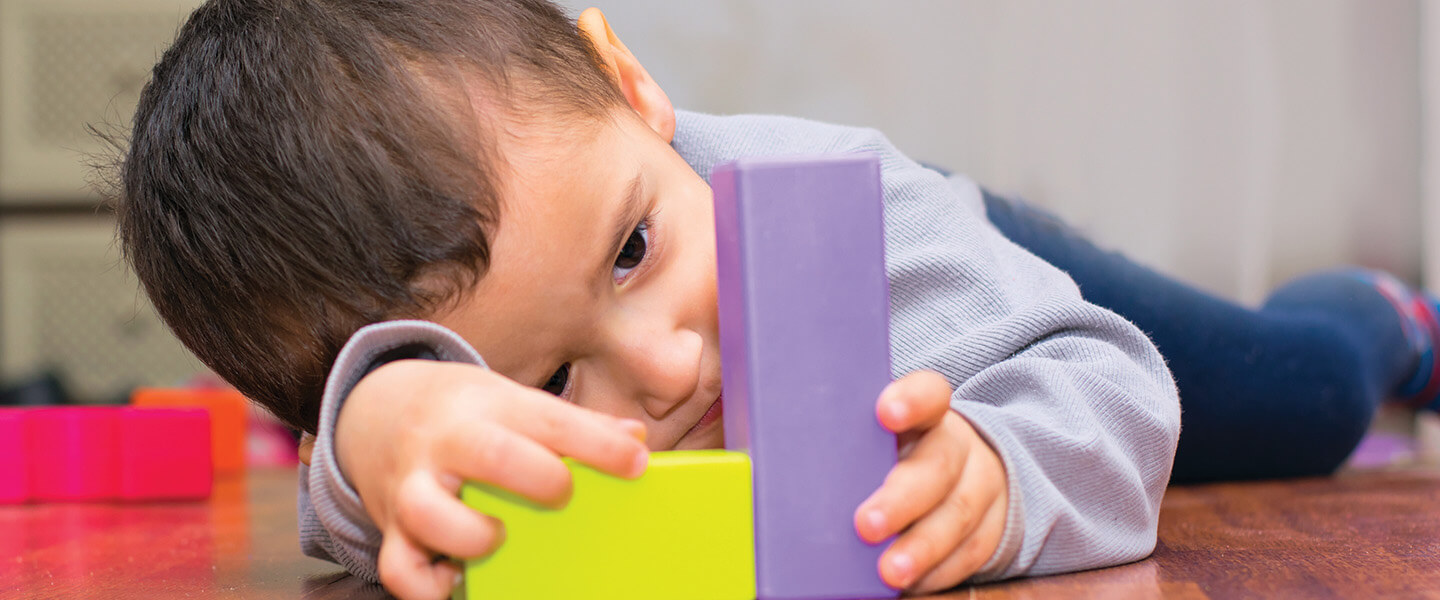
Using a well-understood, inexpensive and easy-to-deploy technology called electroencephalography, or EEG, as well as related technologies that also measure the activity of cells in the brain, researchers funded by BBRF grants are making impressive progress in predicting the likelihood of onset of a number of psychiatric disorders, including psychosis and autism spectrum disorder (ASD).
EEG has also shown promise in indicating the likelihood that individuals with major depression will respond to specific antidepressant treatments. Further, the technology is being used as a discovery tool to reveal underlying pathology, for example in schizophrenia and post-traumatic stress disorder (PTSD).
First demonstrated in a human subject in the 1920s (with a crude version of the technology), EEG measures electrical activity generated by the brain’s neurons. It’s non-invasive, detecting those signals from the surface of the scalp. A modern, highly sensitive EEG typically requires a test subject to wear a cap studded with electrodes which are wired together to form a dense array. Recordings can be made in a matter of minutes.
EEG “readouts” reflect neuronal activation relatively close to the scalp, meaning that billions of neurons of the cerebral cortex are within the reach of its detection. To the layman’s eye, a readout from an EEG session looks like a collection of neatly stacked rows of squiggly lines. Each of these rows is registering neuronal activity in a specific “band,” with different bands corresponding with different speeds of neural oscillation. These oscillations range from very slow (1–3 oscillations per second) to very fast (up to 100 oscillations per second). The different bands are referred to with letters of the Greek alphabet. Delta waves, typically generated during sleep, are the slowest; gamma waves, reflecting brain operations involved in consciousness and perception, are the fastest.
PREDICTING AUTISM
Brain mechanisms involved in causing autism’s symptoms are still poorly understood. Yet certain biological correlates of these mechanisms have come to researchers’ attention. One of these is a relationship between differences in brainwaves and autism pathology emerging in the first 3 years of life.
These brainwave differences—revealed by EEG and seen in comparisons of infants who go on to develop autism compared with those who do not—are now thought to be among “the core features of autism spectrum disorder pathophysiology,” according to a team led by 2017 Ruane Prize winner Charles A. Nelson, Ph.D., and 2016 BBRF Young Investigator April R. Levin, M.D., both of Harvard Medical School and Boston Children’s Hospital.
In a paper appearing in Nature Communications in December 2019, the team reported that it had recruited a cohort of 102 infants at high risk—children with one or more older siblings diagnosed with ASD. Such high-risk children are estimated to have a 1 in 5 chance of developing ASD—a rate about 10 times higher than that in the general population. EEG patterns of these children (of whom 31 ended up developing ASD) were compared with one another and with those of 69 children in the study with low familial ASD risk.
EEGs were performed in the study group every few months beginning 3 months after birth and ending in an assessment at 36 months, by which time ASD symptoms are typically apparent and diagnosis in the clinic is possible.
The team discovered that EEG differences in the children who did go on to receive an ASD diagnosis at age 3 were not only detectable but were clearest during the first year of life.
This early-appearing “signal” was seen particularly in slowoscillation delta waves and in high-oscillation gamma waves. It is good news, Dr. Levin says, that readings during the first year were most predictive of future ASD outcome. It is widely thought that the earlier such children are identified, the better their chances of receiving care that might minimize the impact of the disorder.
“What this research told us was that there is a signal in the EEG, in the brainwaves, that is likely to be helpful to predict who is likely to go on to develop autism,” Dr. Levin says. “Our goal is to eventually move toward developing some sort of clinically relevant biomarker. The dream is that kids will come in to their 2- or 4-month pediatrician well-child checkup and in addition to getting their vaccines they will also get an EEG or something similar, to look at their risk for autism.”
However, Dr. Levin stresses that there is an important ethical question in play. Even if a biomarker predicting a later autism diagnosis is fully validated for clinical use, “you don’t want to be diagnosing a disorder early if you’re not sure that treatments you have at hand are really going to be effective.”
For this reason, she says, “it’s really important to recognize that we’re not yet at a point where we can make clinical recommendations based on the findings in our paper.” Apart from the question of treatments, Dr. Levin adds, the EEG signal needs to be replicated experimentally and optimized, so that it is highly specific to future ASD diagnosis and sensitive enough to minimize the chances of generating false positives and false negatives. She and the team are also working on questions of logistics, she says—how to equip pediatrician offices to provide EEG tests for very young children, and how to implement software that will perform the analysis on-site.
On the research side, Dr. Levin says that she and colleagues are focusing on ways “to learn more from the millions of data points” generated even in a 5-minute EEG. “We’re trying to pull out more information from these data points,” with the hope of being able to know more about the mechanisms underlying the patterns so far detected, particularly in infants who go on to develop ASD.
PREDICTING ANTIDEPRESSANT RESPONSE
Researchers using EEG have taken an important step toward objective biology-based markers on which to base depression treatment decisions.
Amit Etkin, M.D., Ph.D., a 2012 BBRF Young Investigator at Stanford University, and Madhukar Trivedi, M.D., a 2002 BBRF Independent Investigator and 1992 Young Investigator at the University of Texas Southwestern, led an international team that identified a brain-wave signature which enabled them to “robustly predict” whether depression patients would respond or fail to respond to the antidepressant sertraline (Zoloft).
The signature also enabled them to compare and distinguish “responders” to sertraline with other patients who responded to a different form of antidepressant therapy, the non-invasive brain stimulation method called repetitive transcranial magnetic stimulation (rTMS).
The team used a resting-state EEG, which measures brain waves while an individual is not engaged in a particular task. The signature they identified was discovered with help from a computer-driven machinelearning program called SELSER. The team’s dataset was derived from four separate studies.
Multiple data sets enabled the team to develop a theory of what their EEG signature in “responders” to sertraline signified about activity in the brain. In a 2020 paper published in Nature Biotechnology, they proposed that better response to the drug correlates with greater excitability in the prefrontal cortex, compared with that in poor responders.
Drs. Etkin, Trivedi and colleagues also made an intriguing observation about responders to sertraline vs. responders to rTMS brain stimulation treatments. Here they saw an inverse correlation: individuals who responded to one of the two rTMS protocols that were tested were less likely to be sertraline responders, and vice-versa.
An important question still to be answered about the potentially predictive EEG signature is whether it is specific to sertraline, or to the larger class of SSRI antidepressant medicines that includes sertraline, or if it has even broader applicability in predicting responses to other antidepressant therapies including electroconvulsive therapy (ECT) and psychotherapy. Dr. Etkin says he and colleagues are currently engaged in studies designed to answer such questions.
The research team also included: Maurizio Fava, M.D., 1994 BBRF Young Investigator; Myrna Weissman, Ph.D., BBRF Scientific Council member, three-time BBRF grantee, 2020 Pardes Prize winner and 1994 Selo Prize winner; Patrick McGrath, M.D., 2002 BBRF Independent Investigator; Thilo Deckersbach, Ph.D., 2004 and 2001 BBRF Young Investigator; and Gregory Fonzo, Ph.D., 2019 BBRF Young Investigator.
PREDICTING PSYCHOSIS
Predicting which individuals considered to be at high risk of developing psychosis will in fact go on to develop psychosis—thus potentially making early or preventive treatment possible—has long been among the objectives of neuropsychiatric research. The challenge is this: only a minority of individuals at clinical high risk will develop psychosis within 4 years. (A recently published analysis put the figure at 22%.) Can they be identified in advance?
High-risk individuals include those with a family history of psychotic disorder, since a significant portion of the risk is thought to be genetic. Other factors include the appearance of what doctors call “sub-threshold symptoms,” which may include brief episodes of distorted thinking, paranoia, delusions, or hallucinations. High-risk individuals may also experience a decline in their ability to function socially.
Peter J. Uhlhaas, Ph.D., of Charité Universitätsmedizin, Berlin, Germany, used his 2009 BBRF Young Investigator grant to explore the hypothesis that imprecise timing of neural activity—potentially detectable in brainwave signatures—is among the core aspects of schizophrenia pathophysiology. In a 2020 paper published in JAMA Psychiatry, Dr. Uhlhaas, with co-authors including 2009 BBRF Distinguished Investigator Stephen Lawrie, M.D., reported that the timing of high-frequency oscillations in visual cortices of the brain “is the first impairment to emerge” in individuals at clinical high risk of first-episode psychosis, and that other features of brainwave oscillations may predict future clinical course.
The team recruited 232 participants, most of them in their late teens or twenties: 119 met criteria for being at clinical high risk (CHR) of psychosis; 38 did not meet these criteria but had been diagnosed with non-psychotic psychiatric disorders; 26 had experienced first-episode psychosis (FEP); and 49 were healthy controls. All were regularly evaluated over a 3-year period. And all were given, at baseline, a task to perform on a computer which required them to press a button in response to visual stimuli on the screen that varied in duration from ¾ of a second to 3 seconds.
While participants performed 3 blocks of 80 such tests, their brainwaves were monitored using magnetoencephalography (MEG), which records magnetic activity of the brain (as distinguished from EEG, which measures electrical activity). MEG, which like EEG is non-invasive, is performed by placing a magnetic coil just above the test subject’s head. MEG is better than EEG in identifying locations in the brain where the waves it detects are generated.
Results of the trial, published in JAMA Psychiatry in April 2020, corroborated past research highlighting the importance of the visual cortex in cognitive processing in the healthy brain—and offering new evidence suggesting how specific aberrations in brain waves generated by neural activity in the visual cortex relate to cognitive deficits seen in FEP, schizophrenia, as well as, in certain respects, in those at high risk of developing FEP.
The team demonstrated that in both FEP and those at high risk for it, the “synchrony” of waveforms generated in the visual cortex in two high-frequency bands—gamma waves (up to 100 oscillations per second) and beta waves (up to 40 oscillations per second)—was notably inconsistent. Importantly, this new research shows that such inconsistencies are present in people with pre-psychosis symptoms.
The research also revealed that in both FEP participants and those at high risk of psychosis, connectivity between the frontal portion of the brain and occipital areas containing the visual cortex was impaired; and that in contrast to participants at high-risk, those who already had a first psychotic episode displayed reduced power in high-frequency gamma waves emanating from the occipital lobe.
Yet another important finding from the research was the observation that those at high risk for psychosis who had displayed increased variability of certain waveform patterns between different “runs” in the visual processing test went on to have more persistent “sub-threshold” symptoms of psychosis—an indication that they would have poorer clinical courses and increased likelihood of progressing to a first psychotic episode and perhaps also schizophrenia.
“Impaired high-frequency oscillations in the visual cortex are an important aspect of circuit dysfunction, which could constitute a biomarker for clinical staging of emerging psychosis,” Dr. Uhlhaas and colleagues concluded. Their future research will focus on circuit mechanisms regulating neural responses, “which may offer targets for preventive approaches.”
PROBING PTSD
Pioneering research in recent years has suggested linkages between the presence of PTSD symptoms and specific changes in brain connectivity. Stanford University’s Amit Etkin, M.D., Ph.D., in addition to his research described above in using EEG to predict antidepressant response, has sought to translate fMRI imagingbased insights on changes in brain connectivity in PTSD patients to a technology that would be easier to use in the clinic, where it might help combat vets and others with the disorder. The results of their latest research appeared last year in the American Journal of Psychiatry.
Dr. Etkin and colleagues first tested their EEG-based technological package on 36 healthy subjects, demonstrating its ability to make fine distinctions in brain connectivity across different brain regions. The team’s solution was based upon resting-state EEG brainwave readings made when the brain is not engaged with a specific cognitive task.
This preliminary test enabled them to piece together a fine-grained “connectomic profile” of brain regions implicated in PTSD—a portrait of how different regions connect with one another. The key question was whether this method would enable the team to see changes in connections that fMRI studies have suggested may be occurring in PTSD. Assuming they were able to do so, the team also wanted to try to relate such connectivity changes with actual symptoms experienced by combat vets with PTSD.
Dr. Etkin’s team next tested their EEG-based method in a group of 201 veterans who had been deployed to Iraq and Afghanistan: 95 were healthy controls without PTSD, while 106 met full or “sub-threshold” criteria for PTSD. Most were men.
The trial revealed 74 brain region connections that were “significantly reduced” in PTSD. The most prominent area of under-connectivity was in a large section of the frontal lobe called the middle frontal gyrus. This underconnectivity was seen in slow-moving theta waves. Such slow waves are associated with memory, emotion, and sensation. These findings were consistent with findings made in prior fMRI studies.
The team was also able to correlate the observed under-connectivity changes with symptoms in patients, involving working memory and inhibition. The team, which is currently engaged in studies extending the work, hopes that validation of the EEG-based method of analyzing brain connectivity in PTSD patients might support clinical efforts to develop more targeted and effective treatments. These could include noninvasive brain stimulation technology (such as repetitive transcranial magnetic stimulation, or rTMS) to target specific brain areas to boost connectivity where it is reduced—it is hoped, with therapeutic impact.
INSIGHTS ON SCHIZOPHRENIA
A research team led by Gregory A. Light, Ph.D., a 2013 BBRF Independent Investigator and 2006 and 2003 Young Investigator at the University of California, San Diego, early this year reported using resting-state EEG to measure brain waves across a broad spectrum of frequencies in 139 schizophrenia patients and in 126 unaffected people who served as controls.
Their results, published in Frontiers in Psychiatry, identified widespread patterns of hyperconnectivity in schizophrenia patients compared with controls, in networks that involved the frontal, temporal, and occipital regions of the brain.
Specifically, the team identified two primary abnormalities in restingstate networks. Among the potential implications of these results, Dr. Light and colleagues noted, were that “patients may show abnormal excessive simultaneous activation of various perception-related brain regions.” This abnormal activation, they said, “may ultimately contribute to clinical symptoms such as hallucinations and delusions” as well as problems assessing the salience, or relative importance, of incoming sensory information.
The researchers hope to confirm the observed brain-wave patterns and relate them to specific pathological processes in schizophrenia. Among other things, they said, it would be important to study whether the observed EEG patterns can also be seen in high-risk individuals prior to disease onset, as well as in those who have recently experienced a first episode of psychosis.
If validated in future studies, the team said, the resting-state EEG patterns they detected might be useful as biomarkers for schizophrenia.
The team also included David L. Braff, Ph.D., 2014 BBRF Lieber Prize winner and 2007 BBRF Distinguished Investigator; and Yash Joshi, Ph.D., a 2018 BBRF Young Investigator.
Written By Peter Tarr, Ph.D.
Click here to read the Brain & Behavior Magazine's May 2021 issue