Team Tests Model to Enable More Effective Targeting of Non-Invasive TMS Treatments in Depression
Team Tests Model to Enable More Effective Targeting of Non-Invasive TMS Treatments in Depression
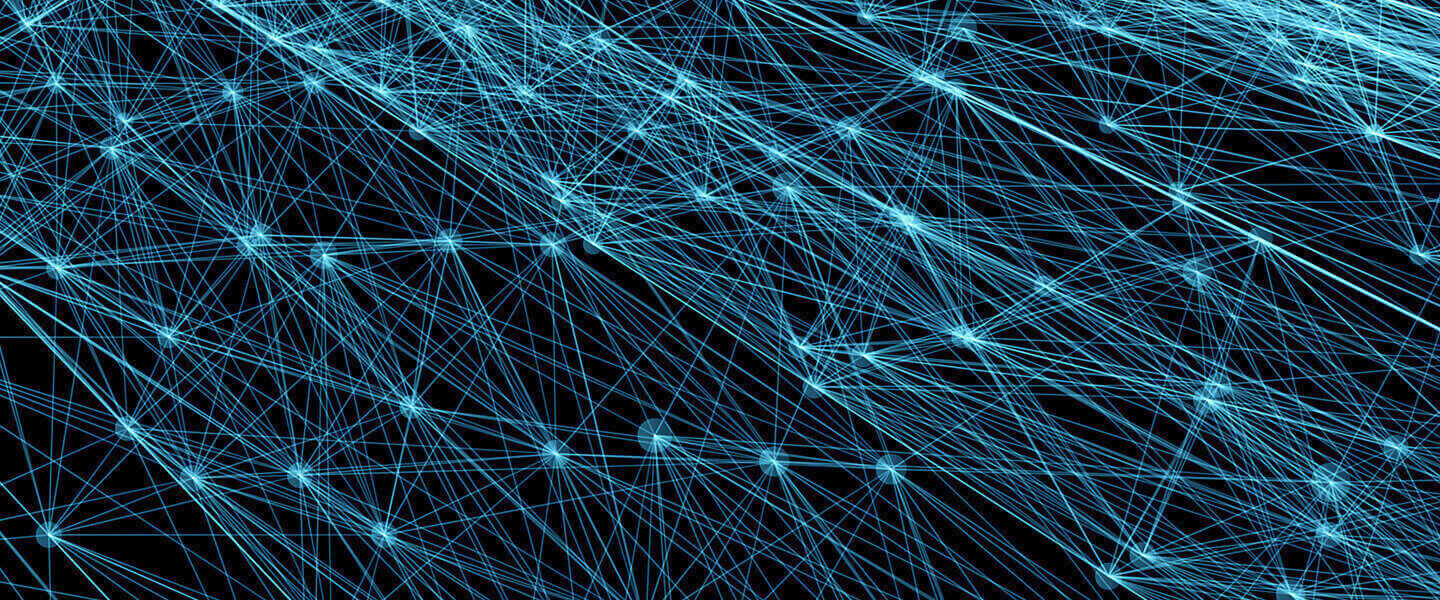
TMS (transcranial magnetic stimulation), a form of non-invasive brain stimulation used to treat depression, has shown its ability to reduce symptoms in many thousands of patients in the years since its approval by the FDA in 2009. TMS is sometimes recommended when a patient doesn't respond to antidepressant drug therapy, although it can also be used as a first-line therapy. Like all anti-depressant therapies, it is more effective for some patients than others.
In recent years, a number of researchers have aimed to discover ways of more precisely targeting TMS to achieve greater efficacy. This research has been inspired, among other things, by evidence indicating that the stimulation delivered in conventional TMS treatments likely affects areas of the brain beyond the area immediately targeted. Indeed, some of the beneficial effects of TMS may depend on such "off-target" effects.
Now, Nicholas L. Balderston, Ph.D., a 2021 BBRF Klerman Prize winner and 2018 BBRF Young Investigator, and a team at the University of Pennsylvania have reported results of a proof-of-concept test of a new method of targeting TMS. The team's senior member was Yvette I. Sheline, M.D., a member of BBRF's Scientific Council and a 2005 and 2002 BBRF Independent Investigator and 1998 Young Investigator.
Drs. Balderston, Sheline and colleagues developed a sophisticated model intended to predict the impact of TMS therapy in specific patients under specific treatment conditions. The aim is to use the model, if validated, to find the best spot in the brain to target and deliver TMS in a given patient, in order to achieve maximum reduction in depression symptoms.
The math-based model, which reflects data about neural connectivity and electric fields in the brain, seeks to calculate the relationship between symptoms and connectivity in each depressed patient, as well as the expected effect of TMS upon connectivity.
The model is built upon data about neural connectivity gathered from across the brain in resting-state functional connectivity (rsFC) scans in depressed individuals. This data generates an initial prediction about the impact of TMS treatments. This is then "augmented" by information about the distribution of electric fields across the cortex, reflecting different targeting sites and different orientations of the equipment delivering TMS stimulation relative to these targets.
The team's model was tested at two broad TMS target areas, one in the left dorsolateral prefrontal cortex (DLPFC), the other in the motor cortex. The left DLPFC is a standard stimulation target in TMS; the motor cortex is not a therapeutic target and was used as a control.
The model was applied to data drawn from a patient cohort composed of 91 "anxious misery" (AM) patients, among whom were individuals with several psychiatric diagnoses including depression (50), anxiety (26) and PTSD (17). The cohort also included 25 healthy controls. The typical study subject was about 29 years old. The test did not involve delivering TMS treatments, but rather predicting the impact of such treatment on each member of the cohort, using a database that included results of two functional MRI scan sessions that each received. These scanning sessions yielded structural diffusion, resting-state, and task-based data for each individual.
When applied, the team's model predicted that TMS stimulation targeting the DLPFC would lead to significant reduction in depression symptoms in the AM patients in the cohort, but not in controls. This reflects what prior clinical experience has clearly established. Further, the model could predict the magnitude of the anticipated therapeutic effect in each individual, as reflected in standard scores used to clinically assess depression. Importantly, the model predicted that symptom reductions would vary in individuals in a predictable way relative to the exact location of the site targeted within the DLPFC and the orientation of the equipment delivering the stimulation relative to the target.
The team found that the model worked at the level of individual participants as well as the entire group, which suggested to them that their approach "has the ability to identify subject-specific TMS targets to maximize symptom reduction."
One factor that may have contributed to the predictive ability of their model, the team said, is their incorporation of "whole-brain" functional connectivity data. This may help the model register the fact that changes in functional connectivity in the brain following TMS treatment for depression "can be seen throughout the brain in regions that are important for affective responding." Such regions include the insula and the subgenual anterior cingulate cortex (sgACC). Evidence of this suggests that TMS's "net effects on symptoms may be driven by these large-scale network connectivity changes."
The team suggested that if validated, their data-driven targeting approach might be used not only to individualize targeting of TMS at the level of individual patients, "but could potentially be extended to individualize targeting at the single-session level, where each TMS session in a course of treatment is targeted at a specific symptom or set of symptoms, thereby maximizing therapeutic effect."
Drs. Balderston, Sheline and colleagues were careful to note the main weakness of their study: it did not involve actually administering TMS to patients and measuring pre-/post-treatment changes in symptoms. But with publication of their findings in the journal Neuropsychopharmacology, they expressed the hope that other researchers will now test the predictions generated by their model in actual patients in clinical research settings.
The team also included: Desmond J. Oathes, Ph.D., 2016 BBRF Young Investigator; and Zhi-De Deng, Ph.D., 2017 BBRF Young Investigator.