Researchers Train Computers to Identify a Biological Signature of Bipolar Disorder
Researchers Train Computers to Identify a Biological Signature of Bipolar Disorder
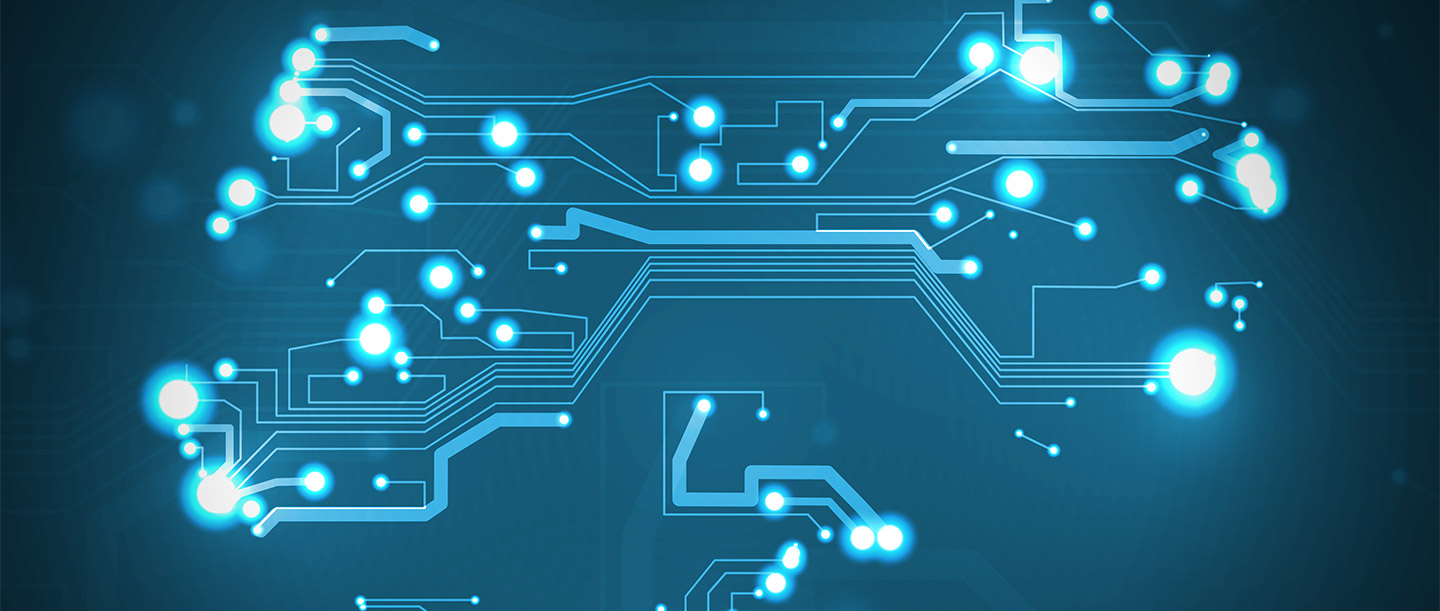
Researchers continue to make progress in their search for biological markers of mental illness. Such markers, if they can be scientifically validated, are widely expected to make diagnosis and risk assessment more objective.
Now, a large international team led by 2015 BBRF Independent Investigator and 2007 Young Investigator Tomas Hajek, M.D., Ph.D., of Dalhousie University, Halifax, Nova Scotia, has reported encouraging results of an effort to use machine-learning methods to identify diagnostic markers of bipolar disorder. The findings are based on information gathered via MRI brain imaging. The report appeared August 31, 2018 in Molecular Psychiatry.
For now, diagnosis of all mental illness continues to be based on observed and reported patient symptoms. Bipolar disorder presents a particular diagnostic challenge, since in many cases it first manifests as depression. A small percentage of depressed people will at some later point experience what is called a manic episode, marked by symptoms that are seemingly diametrically opposed to those of depression: a high state of arousal, great energy, a reduced need for sleep, and a tendency to make rash and impulsive decisions. This subset of patients is considered to have bipolar disorder.
Further complicating the diagnostic task is the fact that bipolar disorder is what scientists call “heterogeneous,” meaning that there are several subtypes with distinct symptom patterns and underlying neurobiology. Therefore, basing the diagnosis on objective biological measures, such as those involving brain imaging, would be very helpful to doctors and patients alike.
Dr. Hajek and colleagues wanted to determine if MRI imaging data could reveal a set of biological properties in the brain that signaled the presence of bipolar disorder in most patients. To test the concept, the team brought together MRI scans from a total of 853 patients with bipolar disorder and 2,167 controls. These subjects were part of a data set in a project called ENIGMA, which has culled patient information from different sites to form large samples. Large samples are required to effectively employ machine-learning methods, in which computers train themselves to identify – in this case – aspects of brain anatomy that correspond with bipolar disorder. Until now, no samples of comparable size had been assembled to do this.
Considering the variability of the illness and the fact that patients for this study were recruited without any coordination in 13 different sites, the researchers were initially doubtful they could differentiate patients from controls based on brain structure. Yet, they emerged with biological parameters from the MRI scans enabling them to identify bipolar disorder patients with about 65% accuracy. “These findings provide a proof-of-concept for a generalizable brain-imaging signature of bipolar disorder which can be detected in individual subjects even within a large, multi-site sample,” the team noted. “Attempting to differentiate patients from controls is the first step before moving to more clinically relevant problems, such as differential diagnosis” -- being able to distinguish between different psychiatric disorders with similar manifestations, they added.
To serve as a clinical diagnostic tool, machine learning will have to generate a diagnostic accuracy of at least 80%. Having access to the actual MRI scans, not just to information about certain brain regions, might considerably improve the performance, said Dr. Hajek. Currently, however, sharing of the full scans entails legal and patient privacy complications, he noted.
Other BBRF grantees participating in the research included: Geraldo Busato, Ph.D., 2010 Independent Investigator; Dara Cannon, Ph.D., 2006, 2004 Young Investigator; Janice Fullerton, Ph.D., 2007 Young Investigator; David Glahn, Ph.D., 2014 Independent Investigator, 2003, 2005 Young Investigator; Roshel Lenroot, M.D., 2003 young Investigator; Colm McDonald, Ph.D., 2009 Independent Investigator, 2002 Young Investigator; Theodore Satterthwaite, M.D., 2014 Klerman Prizewinner, 2010 Young Investigator; Jair Soares, M.D., Ph.D., 1999, 1997 Young Investigator; and Eduard Vieta, M.D., 2012 Colvin Prizewinner.