A Machine-Learning Tool That Predicts PTSD Risk Before Soldiers Are Deployed
A Machine-Learning Tool That Predicts PTSD Risk Before Soldiers Are Deployed
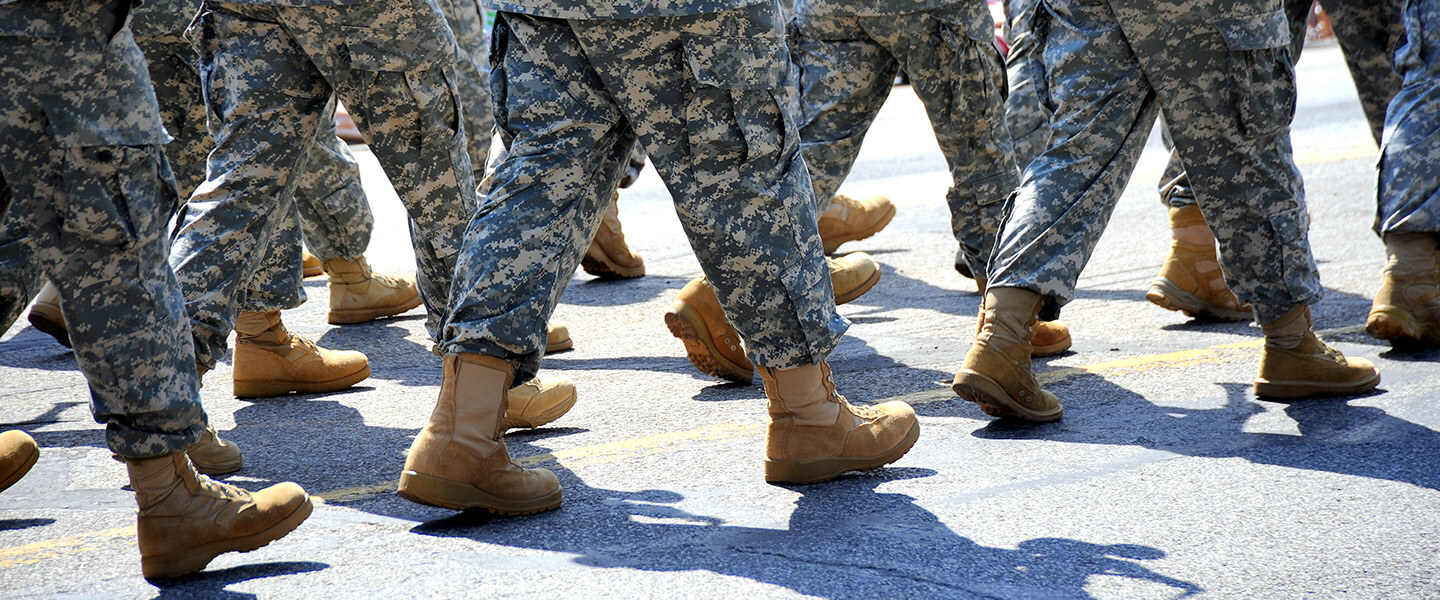
Researchers have successfully tested a new method of predicting, prior to deployment, which soldiers serving in a combat zone are at greatest risk of developing PTSD in the months following their return home.
Gauging risk in advance of deployment could be valuable for several reasons. First, it is known that PTSD can become chronic if untreated and that it is strongly associated with various psychiatric comorbidities and suicide. Developers of the new predictive tool, in a paper reporting their results appearing in JAMA Network Open, suggest that “efficient assessment of PTSD risk among those likely to experience trauma, such as soldiers, may facilitate development of targeted preventive or early interventions that reduce individual suffering and societal costs.”
The research team, led by BBRF Scientific Council member Murray B. Stein, M.D., M.P.H., of the University of California, San Diego, and Santiago Papini, Ph.D., now at the University of Hawaiʻi at Mānoa, used a dataset of 4,771 soldiers from three U.S. Army brigade combat teams based in different parts of the country who were about to be sent to Afghanistan in 2012. Participants were assessed 1-2 months prior to their departure and again, twice, 2-3 and 8-9 months after they returned home. They served in the combat zone for about 10 months.
Almost 95% of the cohort was male and 72% identified as White; the average age was about 27. Post-return assessments revealed that 15.4% of the cohort (746 soldiers) were suffering from PTSD. The question for the researchers was: which of several machine learning-based models of risk prediction that they had developed worked best to identify the soldiers who were diagnosed with PTSD following their return. The sole basis of the various models tested by the team was data collected in the initial assessment, made just before the soldiers deployed for Afghanistan.
The initial assessment, therefore, was critical. Participants completed a self-administered computerized assessment which included in all, some 801 potential pre-deployment predictors of vulnerability to trauma. Some of these factors, if considered alone, would be considered “weak predictors,” although in the context of the full assessment may have had a strong predictive value. Questions asked of the participants pre-deployment included: assessment of symptoms of major depression, mania/hypomania, panic disorder, generalized anxiety disorder, ADHD, intermittent explosive disorder, and substance-use disorders. Suicidal thoughts and behavior were also assessed. Additional measures assessed childhood adversity and misconduct, lifetime trauma, six current and lifetime PTSD symptoms, previous deployment experiences, stress, coping styles, demographics, physical health, injuries, mental health treatment history, weapons ownership, social networks, religiosity or spirituality, and personality.
The machine-learning model ultimately chosen by the team was developed using data from members of two of the brigades within the total cohort and was tested on those in the third to provide an honest assessment of how well the model would perform with data that was not used to develop it. The post-deployment window of assessment, beginning at 2 months after return and ending 9 months after, was designed to rule out confusing early acute stress with PTSD, as well as to capture most delayed PTSD reactions.
The model ultimately chosen by the team used only 58 (“core predictors”) of the 801 potential predictors in the pre-deployment dataset, which was found to perform with accuracy comparable to a model that used all of the predictors as well as a model based on 196 of the potential predictors.
In the cohort that was used to test the final model, the 1/3 of soldiers with the highest predicted risk accounted for 62% of the PTSD cases actually diagnosed in the 9 months following their return from Afghanistan.
While it is impossible to say that this prediction model would yield similar results in a future war involving other soldiers and a different combat zone and conditions, the team does believe the model “provides valuable information about which items may be most informative for predicting PTSD among soldiers who may be involved in future combat situations.”
It will be important to test the model selected in this study with other cohorts of soldiers deployed to combat zones, particularly those with a larger fraction of female participants. The team also hopes that the cost-effectiveness of their approach will be studied in more detail, as well as the question of determining appropriate thresholds for targeted intervention among individual soldiers who are identified by the model to be most at risk prior to their deployment.