Brain-wave EEG Signature Robustly Predicted Antidepressant Response
Brain-wave EEG Signature Robustly Predicted Antidepressant Response
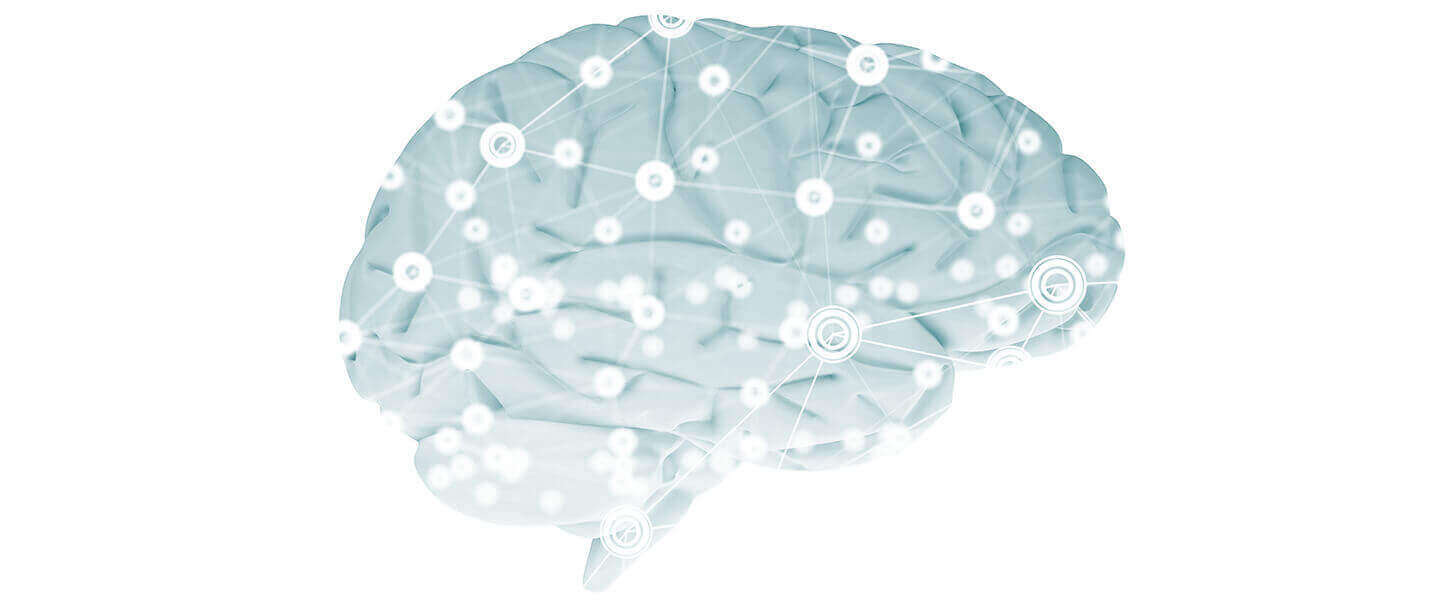
Researchers have reported an important step forward in the search for objective biology-based markers on which to base treatment decisions for depression.
Amit Etkin, M.D., Ph.D., and Madhukar Trivedi, M.D., led an international team that identified a brainwave signature which enabled them to “robustly predict” whether depression patients would respond or fail to respond to the antidepressant sertraline (Zoloft).
The signature also enabled them to compare and distinguish “responders” to sertraline with other patients who responded to a different form of antidepressant therapy, the non-invasive brain stimulation method called repetitive transcranial magnetic stimulation (rTMS).
Dr. Etkin, of Stanford University, is a 2012 BBRF Young Investigator; Dr. Trivedi, of the University of Texas Southwestern, is a 2002 BBRF Independent Investigator and 1992 Young Investigator.
Although these results and their possible implications for personalized depression treatment need to be replicated and extended, the team, reporting in the journal Nature Biotechnology, said that the signature they identified from resting-state EEG (electroencephalography) data “provide[s] neurobiological evidence that an antidepressant-responsive phenotype exists” within the broad spectrum of patients who receive the clinical diagnosis of depression. By “phenotype,” researchers in this context mean a coherent pattern that they can identify across different individuals.
A coherent signal that can be objectively measured and which would accurately predict whether a given person will respond to a specific antidepressant treatment would be a great boon to patients and to medicine more generally. In the absence of fully validated biomarkers, diagnosis of depression and other psychiatric illnesses depends upon a doctor’s analysis of symptoms. For example, one can find in the latest edition of the Diagnostic and Statistical Manual (“DSM-V”) a list of symptoms, five or more of which must be present during the same 2-week period to make a positive depression diagnosis.
The EEG signal reported by Drs. Etkin, Trivedi and colleagues (including 5 other recipients of BBRF grants) is purely biological; it is not, in itself, about behavioral symptoms, but rather about at least one aspect of the underlying brain biology that gives rise to symptoms. The team used a resting-state EEG brainwave signature, which was identified with help from a computer-driven machine-learning program called SELSER.
The team’s data were derived from four separate studies. One was the largest-to-date clinical trial studying antidepressant effectiveness that was randomized, placebo-controlled and was accompanied by neuroimaging of most patients. This study, called EMBARC, involved 309 medication-free outpatients who had been clinically diagnosed with depression and treated with either sertraline or placebo plus psychotherapy over 8 weeks. High-quality EEG data had been collected on 228 of these patients.
This information was used by the team to discover a telltale brain-wave signature, which was then validated by using three additional sets of data. One was a separate group of 72 depressed patients who also had received EEG scans and whose responses to treatment had been assessed; the same EEG signal was predictive in these patients. Another independent group of 24 depressed patients provided data that enabled the team to see if their signature correlated with another machine-learning brainwave signature. A final sample of 152 depressed patients enabled the team to see if their EEG signature was able to predict responses to two variant types of rTMS antidepression treatments.
The team found that the EEG signature they discovered in the first dataset was “generalizable” – it worked well to predict whether a patient would respond to sertraline despite differences in EEG equipment. The other datasets helped the team develop a theory of what their EEG signature in “responders” to sertraline signified about activity in the brain. They propose that better response to the drug correlates with greater excitability in the prefrontal cortex, compared with that in poor responders.
The team also made an intriguing observation about responders to sertraline vs. responders to rTMS brain stimulation treatments. Here they saw an inverse correlation: individuals who responded to one of the two rTMS protocols that were tested were less likely to be sertraline responders, and vice-versa.
Since the EEG signature they discovered could be used to predict response, this suggests that if replicated and validated by others in independent groups of patients, the predictive EEG signal might be a tool doctors could use to determine which form of treatment to prescribe for depressed patients. Failure to respond to a first course of therapy is very common, so this would save not only time and money, but spare patients suffering.
Among the questions still to be answered about the predictive EEG signature is whether it is specific to sertraline, or to the larger class of SSRI antidepressant medicines that includes sertraline, or if it has even broader applicability in predicting responses to other antidepressant therapies including electroconvulsive therapy (ECT) and psychotherapy. It will also be important to test for the signal in patients who have already been exposed to antidepressant therapies; those in the EMBARC trial were not taking antidepressant medicines at the time of their EEG scan.
The research team also included: Maurizio Fava, M.D., 1994 BBRF Young Investigator; Myrna Weissman, Ph.D., BBRF Scientific Council member, 3-time BBRF grantee, and 1994 Selo Prize winner; Patrick McGrath, M.D., 2002 BBRF Independent Investigator; Thilo Deckersbach, Ph.D., 2004 and 2001 BBRF Young Investigator; and Gregory Fonzo, Ph.D., 2019 BBRF Young Investigator.